Evidentiary standards for validation of multifactorial algorithms in Software as a Medical Device (SaMD) diagnostic and predictive signatures.
Diagnostic and predictive signatures, along with their corresponding algorithms, used for patient selection, surrogate biomarkers, and genomic platforms, have been in existence for at least two decades. Genomic signature products began with the availability of DNA microarray. Early predictive signature products included the Genomic Health OncoType Dx signature in oncology (2). These early examples of diagnostic and predictive signatures shared a development path of a rather opaque and proprietary database, signatures, and algorithms.
An additional challenge for their development was the lack of consensus on evidentiary standards for their validation. The continuous changes in these databases, signatures, and algorithms also made rigorous validation at any given point subject to changes in any of these elements. These challenges remain today: there is still no consensus on evidentiary standards for the validation of diagnostic and predictive signatures.
Genomic data platforms identified the need for evidentiary standards early on, but this need applies to any biomarker platform signature. The MicroArray Quality Control (MAQC) consortiums (3) successfully introduced criteria and standard operating procedures during this period to promote reproducibility in the process of developing these signatures. The MAQC publications over the past two decades (4, 5) have become milestones in defining the process of transforming raw data from DNA microarrays, DNA sequencing, or RNA sequencing into genomic signatures and their corresponding algorithms. These articles have shown what is necessary for reproducible signature identification. However, the MAQC stopped short of defining evidentiary standards for the validation of these signatures.
Defining these evidentiary standards is both an important technical task and a regulatory task. In the specific case of genomic signatures, the lack of a clear set of evidentiary standards for analytical and clinical validation has posed an additional barrier to accurately estimating the development costs of these signatures. While “fit-for-purpose” agreements with regulatory examiners help dispel doubts about individual product validation requirements, they also leave some confusion regarding the rationale for different validation studies. A rigorous set of evidentiary standards for signature validation is necessary to approach the level of rigor found in the analytical and clinical validation of other in vitro diagnostics.
Diagnostic and predictive signature algorithms are software products as medical devices (SaMD) (6). The development of SaMD products (7) is supported by several guidance documents (8, 9) published by the FDA over the past decade. These documents generally describe the expected data files to support risk-based 510(k) submissions (market authorization for moderate-risk medical devices) and PMA submissions (pre-market approval for high-risk medical devices) for these products.
The risk categorization framework for SaMD products consists of four categories (I, II, III, and IV) (10), based on levels of impact on the patient. An I-IV risk scale defines the risk for SaMD products. SaMD products must provide accurate information for treatment, monitoring, prognosis or diagnosis, and guiding clinical management. This information may be part of future clinical management of serious diseases such as cancer or Alzheimer’s disease. Whether these diagnostic and predictive signatures include genomic, imaging, or digital measures, these platforms face the challenge of risk-based description of their measurement properties, analytical and clinical validation protocols, and comparison to existing clinical and scientific standards. Rigorous analytical validation of multifactorial diagnostic and predictive signatures would require both individual validation for each component of the proposed signature and for the signature as a whole. Based on statistical, biological, and clinical information, it is likely that more than one signature candidate would be proposed for analytical validation, and this analytical validation process would help select a single signature for clinical validation.
But how do we know if a signature is sufficiently reliable? What are the constraints on these signatures? What is the minimum number of genes they should include? What should these genes be?
Analytical and clinical validation protocols for multifactorial diagnostic and predictive signatures can be precisely written if validated evidence standards are clearly defined. These standards include levels of sensitivity, specificity, and reproducibility for individual components and multifactorial signatures. Analyzing multiple examples of individual diagnostic and predictive signatures across genomic, imaging, and digital platforms will allow for a systematic study of essential validation factors for analytical and clinical validation of these multimodal signatures. These studies will lead to the development of analytical and clinical validation protocols to be used for validating these signatures with data provided by the companies proposing them. A proposal for evidence standards for analytical and clinical validation of these signatures will be possible based on these analyses.
Let’s take the specific case of Ariana Pharma and Bio Rad, who collaborated to develop genomic signatures for early detection of liver fibrosis and identification of individuals likely to respond to combination therapy.
The goal of this collaboration was the development of blood-based diagnostic kits to identify biomarker signatures for early detection of liver fibrosis and individuals likely to respond to combination therapy.
Two US patent were filed for the genomic content of these expression signatures, as well as the molecular mechanisms represented by these genes, in the early detection of liver fibrosis and prediction of treatment response:
US 9624541 B2: Combination of biomarkers for the detection and evaluation of hepatitis fibrosis
US 20130316332 A1: Combination of biomarkers for the prognosis of response or non-response to an anti-HCV treatment
This collaboration highlighted the importance of defining validated evidentiary standards to assess the reliability of multifactorial diagnostic and predictive signatures.
Analyzing multiple examples of individual signatures across different technological platforms will identify essential validation factors for analytical and clinical validation of these signatures. This will lead to the development of accurate validation protocols for data supporting these signatures.
Ariana is a pioneer in the application of artificial intelligence for the identification and optimization of biomarker signatures. It has also become a gold standard for critical validation parameters in the analytical and clinical validation of these multimodal signatures. Ariana plays a major role in both identification and validation of biomarkers. Ariana enables the faster delivery of innovative and higher-quality therapies to patients.
REFERENCES :
1) Ramsay G. DNA chips: state-of-the art. Nat Biotechnol. 1998 Jan;16(1):40-4.
2) Cobleigh MA, Tabesh B, Bitterman P, Baker J, Cronin M, Liu ML, Borchik R, Mosquera JM, Walker MG, Shak S. Tumor gene expression and prognosis in breast cancer patients with 10 or more positive lymph nodes. Clin Cancer Res. 2005 Dec 15;11(24 Pt 1):8623-31.
3) Shi L, Tong W, Fang H, Scherf U, Han J, Puri RK, Frueh FW, Goodsaid FM, Guo L, Su Z, Han T, Fuscoe JC, Xu ZA, Patterson TA, Hong H, Xie Q, Perkins RG, Chen JJ, Casciano DA. Cross-platform comparability of microarray technology: intra-platform consistency and appropriate data analysis procedures are essential. BMC Bioinformatics. 2005 Jul 15;6 Suppl 2(Suppl 2):S12.
4) Canales RD, Luo Y, Willey JC, Austermiller B, Barbacioru CC, Boysen C, Hunkapiller K, Jensen RV, Knight CR, Lee KY, Ma Y, Maqsodi B, Papallo A, Peters EH, Poulter K, Ruppel PL, Samaha RR, Shi L, Yang W, Zhang L, Goodsaid FM. Evaluation of DNA microarray results with quantitative gene expression platforms. Nat Biotechnol. 2006 Sep;24(9):1115-22.
5) Shi L, Jones WD, Jensen RV, Harris SC, Perkins RG, Goodsaid FM, Guo L, Croner LJ, Boysen C, Fang H, Qian F, Amur S, Bao W, Barbacioru CC, Bertholet V, Cao XM, Chu TM, Collins PJ, Fan XH, Frueh FW, Fuscoe JC, Guo X, Han J, Herman D, Hong H, Kawasaki ES, Li QZ, Luo Y, Ma Y, Mei N, Peterson RL, Puri RK, Shippy R, Su Z, Sun YA, Sun H, Thorn B, Turpaz Y, Wang C, Wang SJ, Warrington JA, Willey JC, Wu J, Xie Q, Zhang L, Zhang L, Zhong S, Wolfinger RD, Tong W. The balance of reproducibility, sensitivity, and specificity of lists of differentially expressed genes in microarray studies. BMC Bioinformatics. 2008 Aug 12;9 Suppl 9(Suppl 9):S10.
7) AIML_SaMD_Action_Plan (fda.gov)
8) Software as a Medical Device (SaMD): Key definitions (imdrf.org)
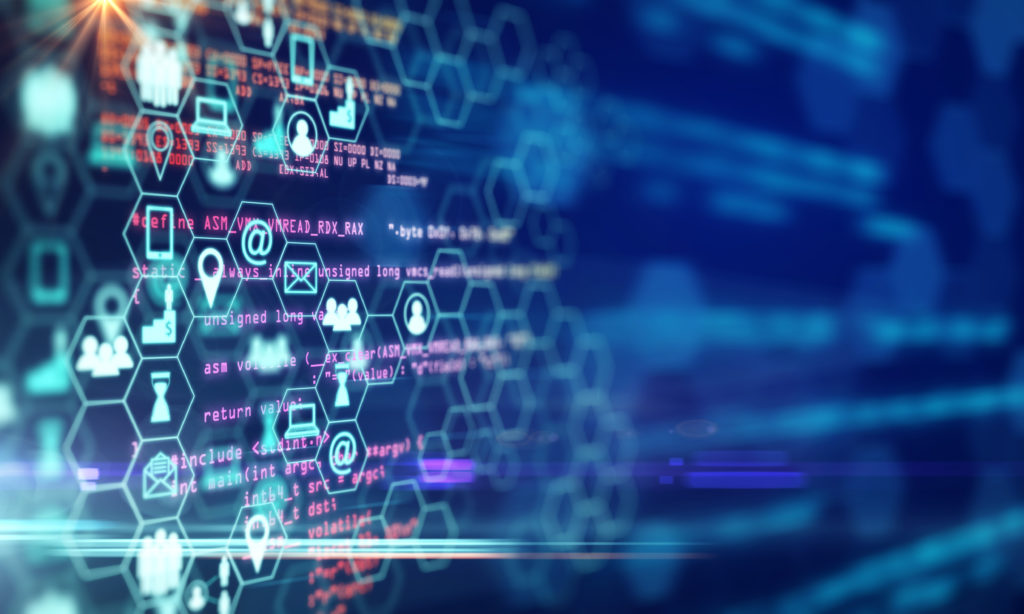
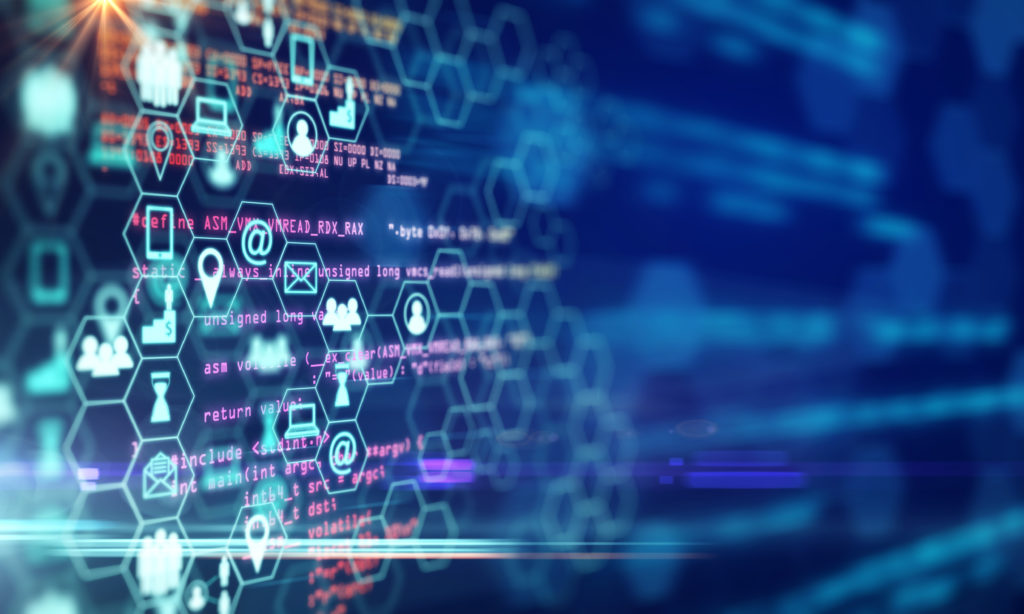